Highlight
Our Department is now recruiting:
– Associate Professor
Our computer laboratory (LSB G25) will be reserved for special activities in the dates below. Hence, it will not be opened for students’ access.
– 25 Apr: 16:00-22:00 (RMSC5102)
– 27 Apr: Full Day (STAT5103)
– 29 Apr: Full Day (RMSC4001)
– 07 May: 12:00-16:00 (STAT4008)
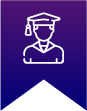
Students Related Affairs
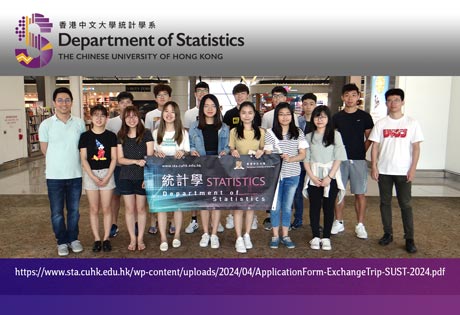
24 April 2024
Student Issues
Exchange Trip to The Southern University of Science and Technology, Shenzhen
View here (pdf) for detailed information.
Download Application Form (pdf).
(Application Deadline: 12:00pm, 7 May 2024)
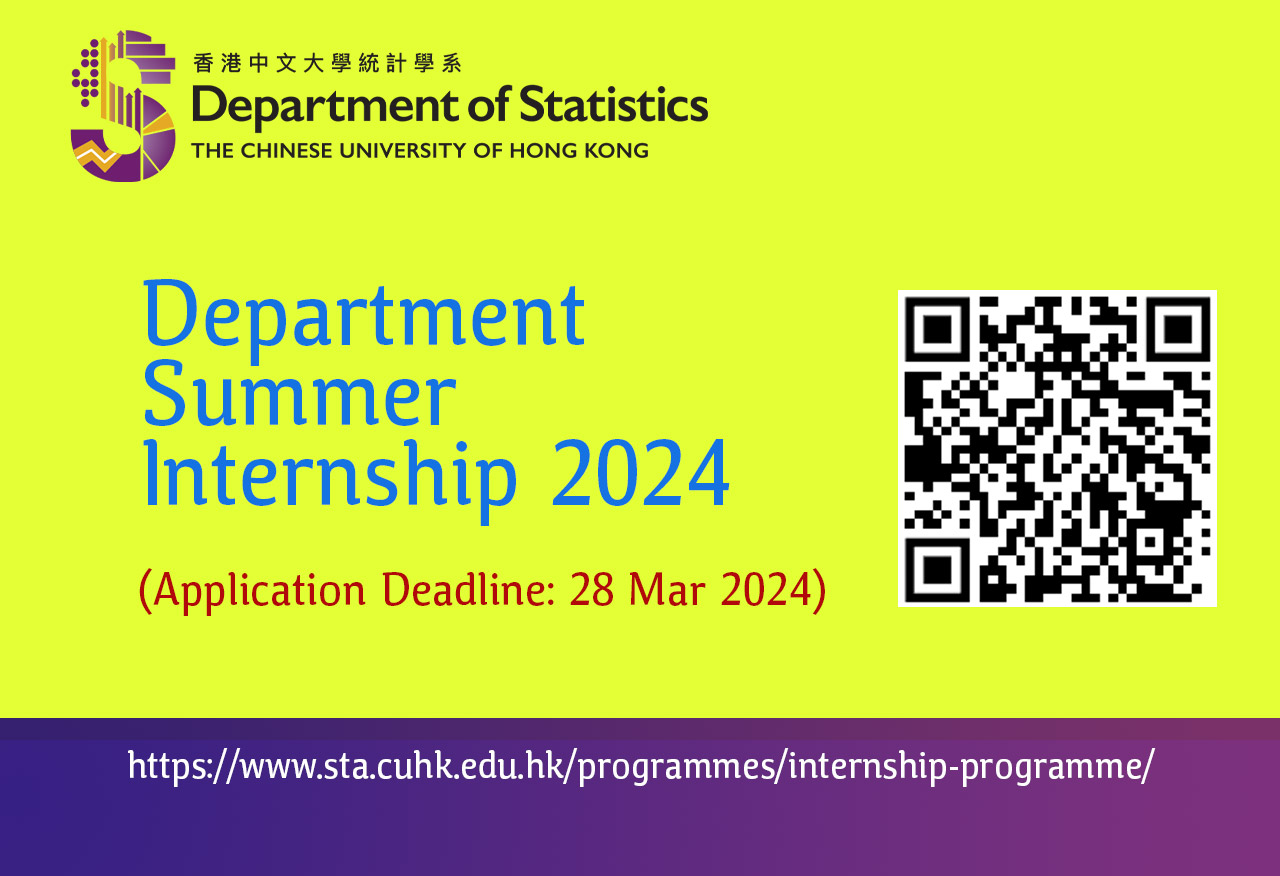
18 March 2024
Student Issues
Department Summer Internship 2024
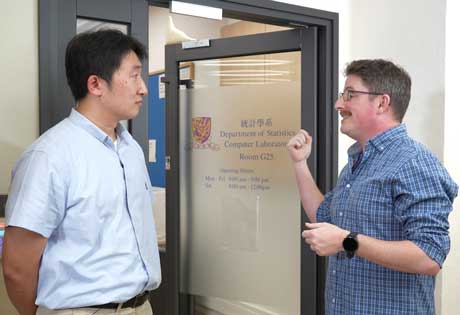
22 February 2024
Student Issues
Resources for Major Students
- Video Introduction to Resources of Statistics Department (MP4/384MB)
- Renovated computer labs;
- Bloomberg terminals;
- Data resources and
- High performance computing clusters.
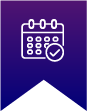
Social Gatherings The Community
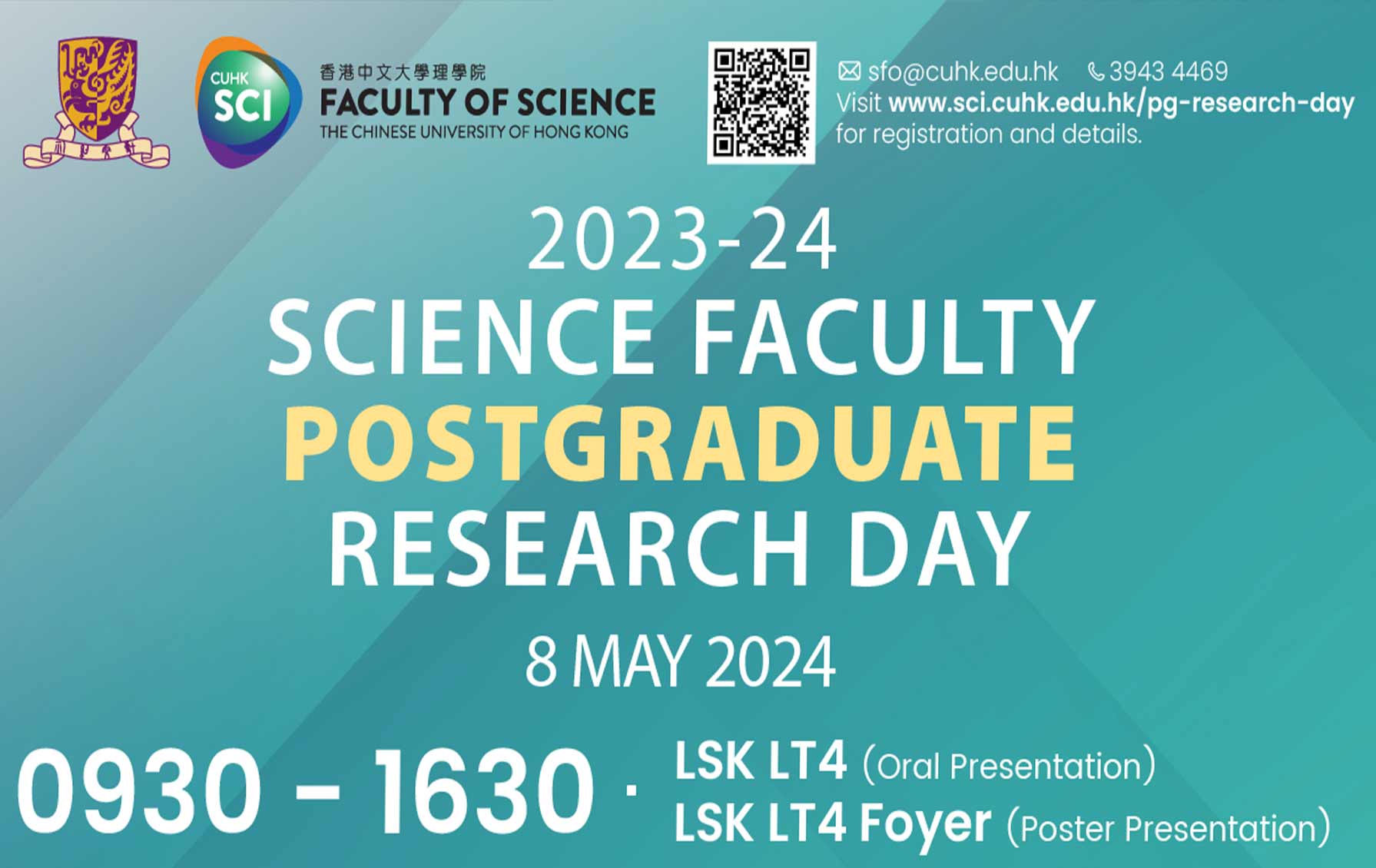
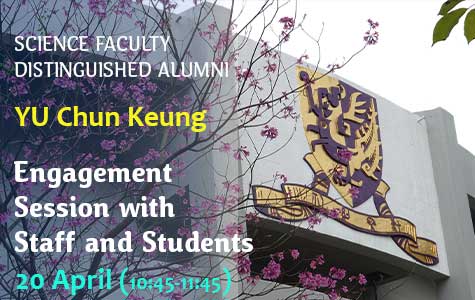
Science Faculty Distinguished Alumni- Engagement Session with Staff and Students
20 April 2024
More Information > LATEST SEMINARS & EVENTS
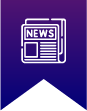
The Latest News of the Department
Undergraduate Studies
Postgraduate Studies
Teacher Quote
Explore More >Alumni Quote
Explore More >Contact Us
Department of Statistics
Room 119, Lady Shaw Building
The Chinese University of Hong Kong
Shatin, N.T.
Hong Kong SAR, China
Get Direction >
Contact Us
Department of Statistics
Room 119, Lady Shaw Building
The Chinese University of Hong Kong
Shatin, N.T.
Hong Kong SAR, China
Get Direction >