Title: Empirical Likelihood Based Inference for Functional Means with Application to Wearable Device Data
Abstract: This talk discusses a nonparametric inference framework for occupation time curves derived from wearable device data. Such curves provide the total time a subject maintains activity above a given level as a function of that level. Taking advantage of the monotonicity and smoothness properties of these curves, we develop a likelihood ratio approach to construct confidence bands and functional-ANOVA type tests to compare mean occupation time curves between groups of subjects. A simulation study shows that the proposed procedures outperform competing functional data approaches. Applications to wearable device data from an NHANES study and an ongoing phase 3 trial of an experimental drug for a mitochondrial DNA depletion syndrome will be discussed.
Based on joint work with Hsin-wen Chang (Academia Sinica). |
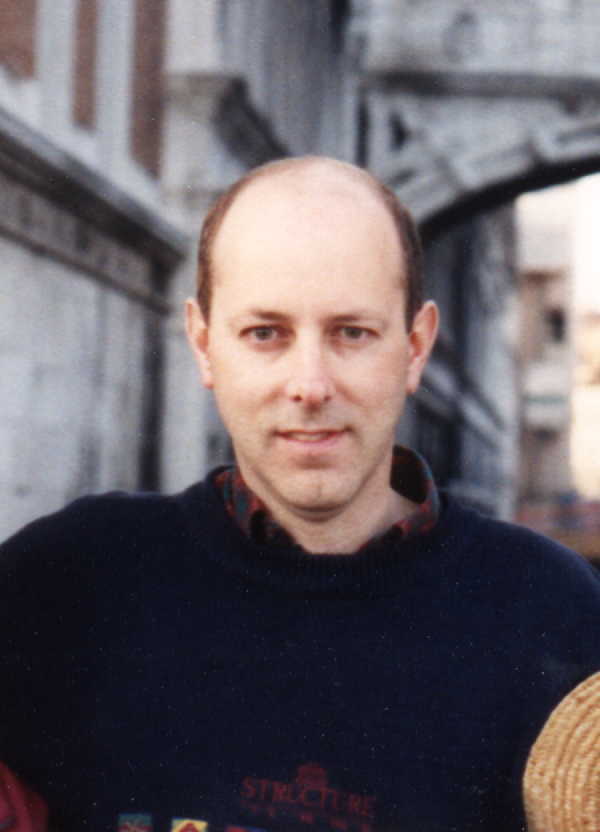
Ian MCKEAGUE
Columbia University
|
Title: Inference for a Large Directed Graphical Model with Interventions
Abstract: Inference of multiple directed relations between primary variables presents challenges in the presence of unspecified interventions. In this presentation, we focus on the problem of inferring multiple directed relations simultaneously while identifying unspecified interventions. First, we derive conditions to yield an identifiable model. Then, we propose constrained regressions for causal discovery to identify the ancestral relations in addition to the instrument interventions for each hypothesis-specific primary variable, eliminating nuisance parameters for hypothesis testing. On this ground, we propose a modified likelihood ratio based on data perturbation to account for the identification effect by perturbing original data to assess the uncertainty associated with identifying ancestors and interventions. For testing the presence and strengths of parent-child relations in a pathway, we show that the proposed tests achieve desired statistical properties. Finally, numerical examples will be given to demonstrate the utility and effectiveness of the proposed procedure.
This work is joint with Chunlin Li and Wei Pan at the University of Minnesota.
Reference: arXiv:2110.03805.
|
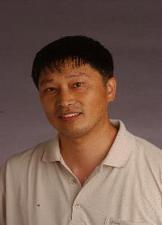
Xiaotong SHEN
University of Minnesota
|
Title: Developing Adaptive Metrics with Relevant Questions from a Questionnaire via Best Subset Algorithms
Abstract: Questionnaire is one of the most widely used instruments to measure variables relevant to certain traits of interest that cannot be easily measured by physical devices. It occurs frequently in practice that the scope for the utility of a questionnaire is beyond the original study population on which the questionnaire was initially developed and validated. In this case, only some items in a questionnaire may be relevant in a new study. We develop a supervised learning method to identify a subset of truly relevant questions to a specific outcome under investigation; in this way, the set of selected items in a questionnaire may be summarized in an optimal way to empower the statistical analysis and improve the interpretation of features or metrics derived from a questionnaire. Our new feature extraction procedure is built upon the best subset algorithm implemented by a mixed integer programming. We establish a large-sample property of selection consistency. This proposed methodology is illustrated by extensive simulation studies and an analysis of the quality of life questionnaire in elderly people with persistent asthma.
This is a joint work with Leyao Zhang, Mengtong Hu and Wen Wang. |
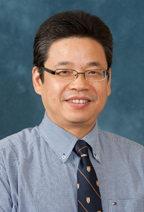
Peter SONG
University of Michigan
|
Title: A Synthetic Regression Model for Large Portfolio Allocation
Abstract: Portfolio allocation is an important topic in financial data analysis. In this talk, based on the mean-variance optimization principle, I will present a synthetic regression model for construction of portfolio allocation, and an easy to implement approach to generate the synthetic sample for the model. Compared with the regression approach in existing literature for portfolio allocation, the proposed method of generating the synthetic sample provides more accurate approximation for the synthetic response variable when the number of assets under consideration is large. Due to the embedded leave-one-out idea, the synthetic sample generated by the proposed method has weaker within sample correlation, which makes the resulting portfolio allocation more close to the optimal one. I will show this intuitive conclusion is theoretically confirmed to be true by the asymptotic properties established. I will also show intensive simulation studies to compare the proposed method with the existing ones, and illustrate the proposed method works better. Finally, I will apply the proposed method to real data sets, and show very encouraging yielded returns.
|
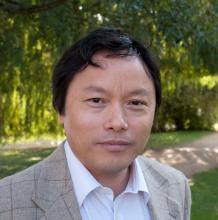
Wenyang ZHANG
University of York
|
Title: Challenges in Biobank-scale Brain Imaging Genetics
Abstract: Recently the UK Biobank study has conducted brain magnetic resonance imaging (MRI) scans of over 50,000 participants. In addition, publicly available imaging genetic datasets also emerge from several other independent studies. We collected massive individual-level MRI data from different data resources, harmonized image processing procedures, and conducted the largest genetic studies so far for various neuroimaging traits from different structural and functional modalities. In this talk, we showcase novel clinical findings from our analyses, such as the shared genetic influences among brain structures, functions, and the genetic overlaps with a wide spectrum of clinical outcomes. We also discuss challenges we have faced when analyzing these biobank-scale datasets and highlight opportunities for future research. This presentation is based on a series of works with members in the BIG-S2 lab of the University of North Carolina at Chapel Hill. Our results can be easily browsed through the Brain Imaging Genetics Knowledge Portal (BIGKP) (https://bigkp.org/)
|
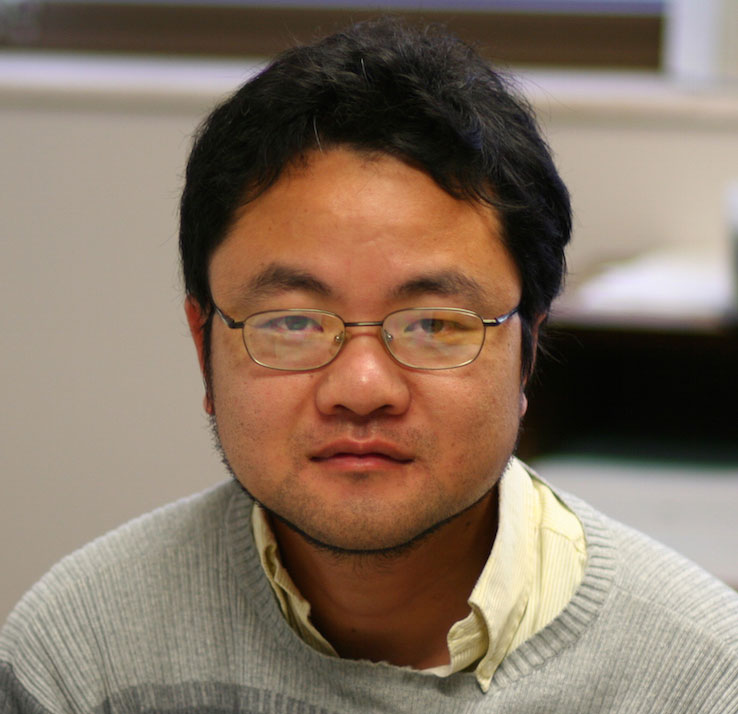
Hongtu ZHU
The University of North Carolina at Chapel Hill
|
|