Title: A Hierarchical Latent Response Model for Inferences about Examinee Engagement in Terms of Guessing and Item-Level Nonresponse
Abstract: In low-stakes assessments, test performance has few or no consequences for examinees,
so that examinees may not be fully engaged when answering the items.
Instead of engaging in solution behaviour, disengaged examinees might randomly guess or omit responses.
When ignored, examinee disengagement poses a severe threat to the validity of results obtained from low-stakes assessments.
Statistical modelling approaches in educational measurement have been proposed that account for non-response or for guessing,
but do not consider both types of disengaged behaviour simultaneously.
We bring together research on modelling examinee engagement and research on missing values and present a hierarchical
latent response model for identifying and modelling the processes associated with examinee disengagement jointly with
the processes associated with engaged responses.
To that end, we employ a mixture model that identifies disengagement at the item-by-examinee level by assuming different
data-generating processes underlying item responses and omissions, respectively, as well as response times associated
with engaged and disengaged behaviour.
By modelling examinee engagement with a latent response framework, the model allows assessing how examinee engagement
relates to ability and speed as well as to identify items that are likely to evoke disengaged test-taking behaviour.
An illustration of the model by means of an application to real data is presented.
|
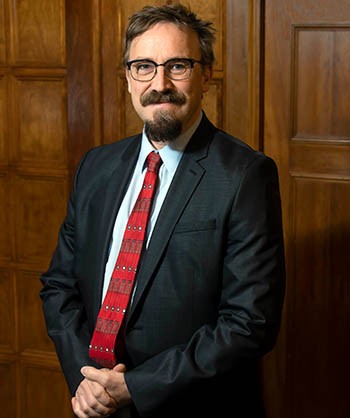
Matthias von DAVIER
Boston College
|
Title: Posterior Inference for Quantile Regression – Adaptation to Sparsity
Abstract: Quantile regression is a well-known approach to study heterogenous relationships in a semiparametric regression framework. Because the sampling variability of the quantile regression estimator generally depends nonparametrically on unknown nuisance parameters, it is often desirable to perform statistical inference without direct estimation of the nuisance parameters. Earlier work has shown that the posterior inference for quantile regression, if properly adjusted, can lead to asymptotically valid frequentist inference. In this talk, we take one step further to show that posterior inference with certain shrinkage priors can adapt automatically to model sparsity, that is, the posterior inference can achieve oracle efficiency for the active coefficients and super-efficiency for the inactive ones in the model. The talk will focus on the asymptotic theory for fixed dimensional quantile regression problems, but will include a discussion how to address high dimensional problems under the same framework.
This talk is based on ongoing work with Yuanzhi Li (PhD candidate). |
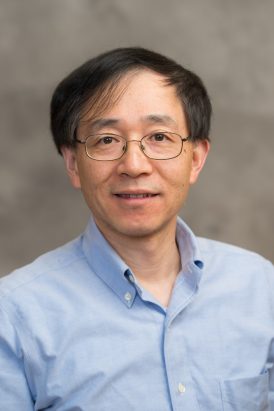
Xuming HE
University of Michigan
|
Title: Consistent Order Selection for ARFIMA Processes
Abstract: Identifying the orders of autoregressive fractionally integrated moving average (ARFIMA) models has been a long-standing challenge in time series analysis. This paper tackles the challenge by establishing the consistency of the Bayesian information criterion (BIC) for the ARFIMA model with independent errors. Because the model’s memory parameter is allowed to be any unknown real number, one salient feature of our result is that it can simultaneously apply to short-memory, long-memory, and non-stationary time series. To further broaden the application range, we generalize BIC’s consistency to the above ARFIMA model with conditional heteroskedastic errors.
We also illustrate the implications of our asymptotic results in finite samples by numerical simulations.
This is joint work with Hsueh-Han Huang, Ngai Hang Chan, and Kun Chen.
|
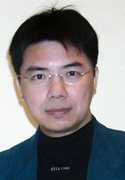
Ching-Kang ING
National Tsing Hua University
|
Title: A Pairwise Pseudo-likelihood Approach for Left-truncated and
Interval-censored Data under the Cox Model
Abstract: Left truncation commonly occurs in many areas and many methods have been proposed in the literature for the analysis of various types of left-truncated failure time data. For the situation, a common approach is to conduct the analysis conditional on truncation times and the method is relatively simple but may not be efficient. In this talk,
we discuss regression analysis of such data arising from the proportional hazards model that also suffer interval censoring and present a pairwise pseudo-likelihood approach that aims to recover some missing information in the conditional methods. Some numerical results will be given and an application to a set of real data arising from an AIDS cohort study will be discussed.
|
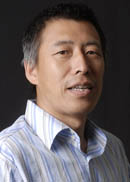
Jianguo SUN
University of Missouri
|
Title: Predicting Disease Risk from Genomics Data
Abstract: Accurate disease risk prediction based on genetic and other factors can lead to more effective disease screening, prevention, and treatment strategies. Despite the identifications of thousands of disease-associated genetic variants through genome-wide association studies in the past 15 years, performance of genetic risk prediction remains moderate or poor for most diseases, which is largely due to the challenges in both identifying all the functionally relevant variants and accurately estimating their effect sizes. Moreover, as most genetic studies have been conducted in individuals of European ancestry, it is even more challenging to develop accurate prediction models in other populations. Furthermore, many studies only provide summary statistics instead of individual level genotype and phenotype data. In this presentation, we will discuss a number of statistical methods that have been developed to address these issues through jointly estimating effect sizes (both across genetic markers and across populations), modeling marker dependency, incorporating functional annotations, and leveraging genetic correlations among different diseases. We will demonstrate the utilities of these methods through their applications to a number of complex diseases/traits in large population cohorts, e.g. the UK Biobank data.
This is joint work with Wei Jiang, Yixuan Ye, Geyu Zhou, Yiming Hu, Qiongshi Lu, and others.
|
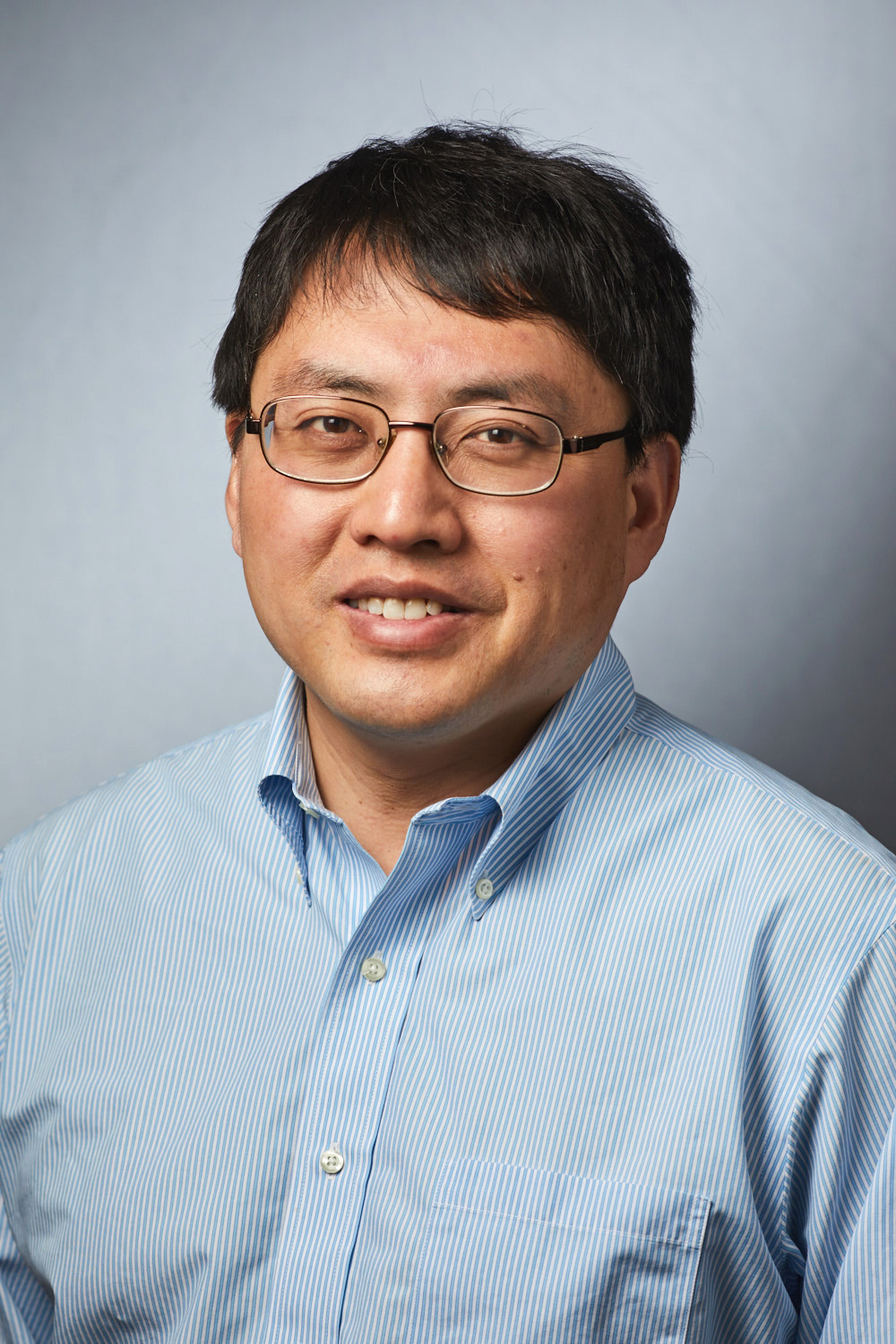
Hongyu ZHAO
Yale University
|
|