HE Xuming
University of Michigan
Title: Post-selection Inference on Regression-based Treatment Effects
A treatment effect often refers to the average causal effect of a binary variable on an outcome variable of interest. The treatment, which could be a medical intervention or a marketing strategy or a policy implementation, is sometimes randomized by design but often self-selected based on individual characteristics of the subjects in the study. To estimate the treatment effect from such studies, statistical adjustments such as regression methods, matching, and use of instrumental variables, have to be used. In this talk, we consider the regression-based methods when many control variables are available. When the inference on the treatment effect is made after model selection, we discuss the challenges from model under-fitting and over-fitting, and argue that (asymptotically) perfect model selection is not the right theoretical framework for statistical inference. We review some of the recent developments in post-selection inference, and highlight some of the key ideas that can be useful in developing valid inference on the treatment effect when a high dimensional control is present. In particular, we examine in detail the idea of aggregation over data splitting and show that it provides a viable method of unbiased inference in high dimensional problems. The talk is partly based on joint work with Jingshen Wang and Gongjun Xu.
|
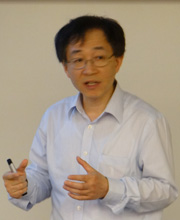 |
TONG Howell
University of Electronic Science and Technology of China /
London School of Economics
Title: Testing for Unit-root Non-stationarity against Threshold Stationarity
- We propose a unit root test for Integrated Moving Average (IMA(1,1)) against the
alternative of a stationary Threshold Autoregressive Moving Average
(TARMA(1,1))[Chan et al., 2017].
- The TARMA framework poses unsolved statistical challenges and requires new
approaches to perform inference.
- The test is based on the recent results on the recurrence properties of the
TARMA(1,1) process [Chan and Goracci, 2017].
- We develop a supremum Lagrange Multiplier test statistic (supLM).
- We derive the asymptotic distribution of the supLM statistic under the null.
- We also propose an unconditional wild bootstrap approach.
|
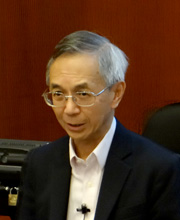 |
TSAY Ruey S.
The University of Chicago
Title: Forecasting in a Data-Rich Environment
We consider and compare different forecasting methods when the data are rich. The methods considered included deep-learning, random forest, and other statistical methods developed for big data. We pay attention to model uncertainty and parameter uncertainty, and discuss various approaches to model averaging and combined forecasts, including Egalitarian Lasso for shrinkage. Real examples are used to demonstrate the analysis and forecasting performance in finite samples.
|
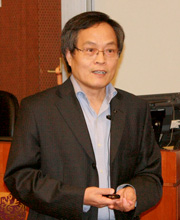 |
YING Zhiliang
Columbia University
Title: Some Statistical Models and Methods for Process Data
This talk presents some recent work on statistical modeling and analysis of problem-solving process data. Such data arise from modern computer-based tests with items for assessing complex problem solving abilities in technology-rich environments. Examples from the Programme for the International Assessment of Adult Competencies (PIAAC) and the Program for International Student Assessment (PISA) will be used to illustrate the new developments.
|
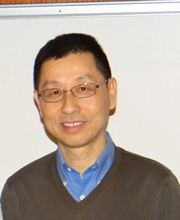 |
|
Last Updated: 22 Nov 2017